Good, Simple Analysis
It's been a few weeks again, I am still in caretaker mode with our first kid. Much respect to the people that spend their time being primary caretakers and also workers, it is so hard to shift mindset from diapers and bottles and enrichment to analytical thinking. Today I’m going to discuss some good analysis.
I’ve written a couple of blogs recently about when data falls short. I recently observed that being a data expert that relies on solid academic and technical foundations is a recipe for becoming a Nihilist, and that’s mostly because you can see where all the seams are in the analyses you come across, most evidently in your own business, but also out in the world of academic studies and news articles. So many decisions are made on bad data, incomplete data, or an incomplete picture of the data. Often the data is overcooked, using advanced statistical and econometric techniques that are unnecessary for the task at hand, but that give the analysis an unearned air of scientific validity which gives it more weight in the eyes of the audience. Frequently the data are not adding value. I don’t think data experts are alone in this. If you do a deep dive into any field, you learn when you get into the weeds that the foundation is put together with a lot of duct tape and wet cement. But occasionally I come across analysis I love. I want to talk about some simple, effective data analysis.
Simple data analysis, built on a basic but rock solid premise, can yield incredible insights. I often preach to my clients that we should strive for simplicity. There is no shortage of data people ready to sell you AI and Machine Learning and any number of Big Data (™) tools that will cost you more than you get from them. Most businesses simply need good, uncomplicated analysis of their data. With that said, here is a chart that I saw recently that is a great example of the form. This is from the Fed.
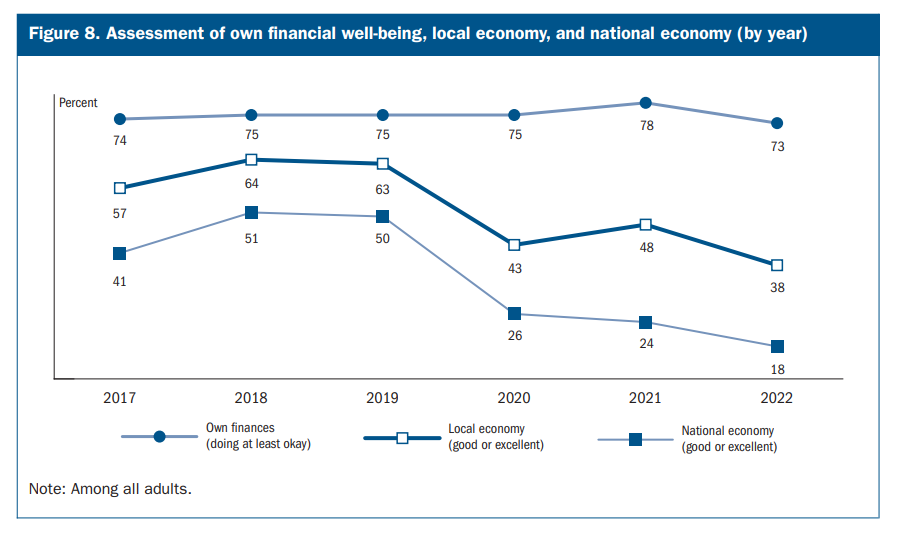
This isn’t the first time I’ve seen this chart, but every time I see it I’m amazed at how many good questions, hypotheses, and observations come out of it. What this chart is saying is that the average American thinks that they are doing ok financially consistently over time. Until 2019 they thought their town was doing pretty well economically, after that it’s iffy, and until 2019 they thought the nation was doing iffy economically, but now they really think the national economy is in the dumps. Setting aside the (somewhat funny) bit where everyone thinks their finances are pretty solid, obviously this scenario can’t be true. If the national economy was in the dumps a bunch of local economies would be doing just as poorly, and many would be doing worse than the nation as a whole. If people were accurately assessing the state of the economy, the local data points would be exactly equal to the national data points as worse local economies are netted out against better local economies all combining into the national average. So what gives?
A couple potential explanations. First, and the one that may be on your mind, is that the way we consume information has become so externalized and polarized that we may actually be in a situation where most Americans think their town is hanging on just fine but the nation is falling apart around them. Another possibility is that people tend to be optimistic about their own situation, and people tend to assess their local situation based on the people around them. It’s unsurprising that people mostly encounter friends and family in the same relative socioeconomic class, and it’s possible that the prevailing feeling about their social group is that “it could be worse”. There are many other possibilities. This chart does the most important thing data analysis can do, it highlights a real insight - that people across the board think their local economy is doing better than the national economy - and allows the data consumers to generate and test hypotheses about why that is the case.
A few notes on what makes this chart so powerful. First, none of these data points are interesting on their own, they are only interesting in relation to each other. I see so much trending analysis that tells you how a number changes over time, but never relates it to an anchor point or another related data point. Trending data without anything to contextualize the trend tends to be meaningless. A stock chart that shows a jump or drop in stock price provides the user with no information, unless the general market trend is included. A stock move could just be in line with the broader market, or could be idiosyncratic to the company itself; the trend line for a single stock does not give you any context. Businesses make this mistake all the time, slide decks are full of numbers that moved up or down with perhaps two or three hypotheses about why the number moved up or down out of hundreds that didn’t make it into the deck. If there was more thought put into the analysis, there might be a second data point that can help contextualize the central data point and empirically reduce the number of possible theories for why a number moved. “Number goes up (or down)” is not a real insight. This FRB chart above is great precisely because it shows the relationship between the average American’s attitudes about their personal finances, the local economy, and the national economy. This helps the user understand that pessimism about the national economy is not entirely due to real world circumstances, there is something else going on.
Second, the methodology is complex but the end user information is simple. This tends to be one of my favorite features of sampling design. It takes effort to define a population, design a sampling frame, decide on your margin of error, and determine how to handle missing values, but the end result is always very simple, easy-to-aggregate statistics with very simple interpretations. Businesses should strive for this in their data analysis. Putting a bit more effort into how you think about the data up front can help improve the insights that come from data analysis, and more importantly, make the analysis repeatable. When the analysis is oversimplified, like the stock movement I highlighted above, then the process of thinking about the universe of potential reasons the stock moved repeats every time the analysis is refreshed. If, instead, a single stock was analyzed alongside the S&P 500, and some sample of stocks from the same industry, now we have a repeatable analysis and some built in explanations for the stock’s movement that yield real insights. None of this is complex statistically, but it is thoughtful. That’s the hallmark of great statistics, simple, but well thought out.
Companies would be better served by not chasing the shiniest new tools and complex data analysis. At the same time, companies should not continue relying on the same old trend lines and percent changes and so on that they see in most of their core business analytics. Instead, companies should think harder about the data they consume regularly. Most core business insights can be improved by adding a thoughtful benchmark, comparative data that seems superfluous on its face, but that can yield great insights, and by relating different areas of the business together that typically aren’t measured together. All of this narrows the set of insights and hypotheses that leaders take away from the data, and improves business responses to new data.