Nate Silver: The Good and Bad
A couple weeks ago it was reported that Nate Silver is out at FiveThirtyEight, the brand he expanded massively on his “perfect” prediction of the 2012 election outcome in all 50 states. I want to talk about Nate Silver, because to me he’s a great example of a data evangelist who, in his earnest effort to fight innumeracy, has a very mixed record. His staunch advocacy for following the numbers and trusting models contributes to the unscientific phenomenon of Scientism. Scientism has infected public discourse from all sides on all manner of topics, beyond the technocrats most associated with the term.
Let’s start with the good. Nate Silver is fully committed to pulling the discourse out of punditry by applying science and statistics to politics (and sports, but I think sports, particularly baseball, was already ahead of the game here). I think he has done a good job of it. He’s helped people better think in terms of the odds of an event happening instead of treading the well worn path of backfilling a narrative around why an event might happen and changing that narrative if events break differently. I’m not sure what kind of audience FiveThirtyEight reached under his leadership, and it’s certainly possible that his most committed readers were the people that already understood statistics on some level, but I appreciated that there was a space for fairly strong technical analysis of the state of political races.
Silver’s analysis is important to understanding, for example, why the backlash against FiveThirtyEight, and pollsters in general, after the 2016 election was fairly unfounded. Some of the complaints about 2016 polling were from partisans greasing the wheels for future election denialism, but there is no doubt that many Americans felt like pollsters got 2016 wrong. There is some truth to that, but the fallacy here is that too many people thought that a poll showing Hillary with a lead in vote share was the same thing as predicting a Hillary victory with a high degree of certainty. Polls can be converted to odds, and the smaller that the poll’s margin of victory is, the lower the odds of victory are. A presidential election poll showing 70% vote share for candidate X against 30% vote share for candidate Y would have very high odds for a candidate X victory, likely a 99+% chance that candidate X eventually wins the election. However, if the poll’s margin was more like 52%-48% those odds would condense, maybe in this case that translates to something like a 60% chance of candidate X winning. These numbers are made up, but this is the general behavior of an estimated polling margin and the odds that a given candidate wins or loses. In many pivotal states that Hillary lost in 2016, the polling margin was very narrowly in her favor, meaning her odds of her winning were better than Trump’s in those states, but not much better. In the 2016 general election, there were some polls and poll aggregators that missed the mark pretty badly, giving Trump a miniscule (basically 0% chance) of winning, but most gave him somewhere between a 1 in 10 or a 3 in 10 chance of winning, which isn’t exactly small. Nate Silver has been banging the drum on this consistently.
I have two complaints about Silver’s coverage, one that I’ll give short shrift and one that I’ll dig into. The first is that his analysis often felt like hiding behind the numbers. I understand that everyone needs a lane, and statistical analysis was his and FiveThirtyEight’s lane, but “I’m simply giving the numbers” is also a way of avoiding taking a stand on an issue. It’s hiding behind the technical analysis on topics that have real moral implications. This has real business analogs, as I noted in my blog on corporate strategy, doing popular things or market tested things is not always the right answer, and experimenting with novel ideas is important. So it is in politics, politicians and ideas that poll well may not be good politicians or good ideas, and orienting coverage solely to the numbers elides the moral question. One caveat I’ll give is that Silver’s staff at FiveThirtyEight would often engage in more pundit-like discussion about topics for which they had hard data, which mitigates this to an extent, but all too often the attitude was “the numbers are what they are” and when that approach is taken to the extreme, it’s a bad approach.
My second complaint is kind of a mishmash of two complaints about modeling. First Silver understands that his models are generating odds, not predictions, but is happy to say that FiveThirtyEight predicted an outcome whenever something happens for which they assigned more than a 50% chance (or in a situation where there’s more than a two-way race, the highest odds). This is his attempt to have his cake (be statistically rigorous) and eat it too (take advantage of innumeracy to raise FiveThirtyEight’s profile). Second, the political models, in particular, are not all that good. These items together, relying on the numbers as gospel, saying they are more powerful than they are, and basing those numbers on over-cooked modeling, are a recipe for the modern invocations of scientism.
Let’s start with Silver’s muddying of the waters on odds vs prediction, because that will lead us right into the quality of the models. Silver and FiveThirtyEight wrote an entire bit of apologia about how they got the 2016 GOP primary wrong, but he spent some throat clearing time extolling the virtues of the data journalist. From the article:
The FiveThirtyEight “polls-only” model has correctly predicted the winner in 52 of 57 (91 percent) primaries and caucuses so far in 2016, and our related “polls-plus” model has gone 51-for-57 (89 percent)
We’ve already discussed why this is a misstatement of what his models are doing, and he knows that’s true. He doesn’t even evaluate his models based on “prediction” he evaluates them on how accurately they portrayed the odds. Let me illustrate by example. Let’s say Silver’s model gives a candidate 55% odds of winning the election. When he is defending data journalism the way he does in his quote, he is saying that the model is predicting that candidate’s victory. However, when he is evaluating his models, he actually (correctly) expects that candidate to lose 45% of the time. The distinction is important, particularly in politics, because predicting election results, for the most part, isn’t all that hard. Correctly evaluating the odds is much harder.
Take a typical house election year. There are 435 House seats, and in a given modern election year maybe 30-40 of those seats are in truly competitive races. This means it’s pretty easy to have a (relatively) high accuracy rate in predicting the winners of the elections. You could likely pull a person off of the street, show that person the by-party vote margin in the prior election cycle, and ask them to predict the results of the upcoming election and they would likely get a minimum of 390-400 seats right. Or, let’s take another example that Silver likes to cite, that he correctly predicted the results in all 50 states in the 2012 Romney-Obama election. We all know that only a handful of states are truly competitive in a given presidential election cycle, so predicting the result of 44-46 states is not all that difficult of a task simply by using the partisan results of the last presidential election. It is harder to pick the toss ups, and some unexpected results may occur, and this is where Silver’s modeling would presumably give them an edge. So let’s see how he does with House elections. The chart at that link is interactive, but I’ll describe some numbers here.
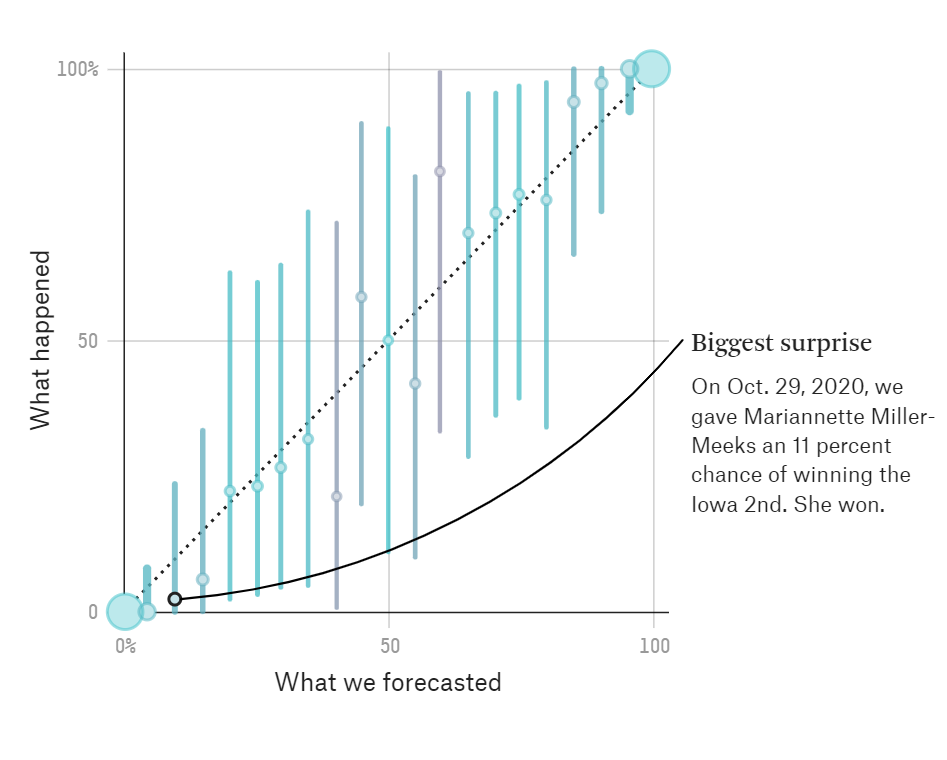
This chart from FiveThirtyEight is a calibration plot. It measures how often an event happens compared to the odds they gave it happening, so if they give an event 50% odds of happening, it should happen about 50% of the time. Each dot and vertical line is a 5% odds increment. The closer the dots are to the dashed line, the better. The size of the dots represents the number of observations they had with that prediction characteristic. The very large bubbles near 100% and 0% are aligned with what we discussed about House elections, most of them are not very competitive and easy to predict, and their model recognizes that, giving the respective candidates in those elections 99% odds and 1% odds of winning. I want to focus on the middle of the range, where presumably statistical modeling would have a serious advantage. The events that they say have 60% odds of occurring happen 81% of the time, and events they assign 40% odds of happening occur 21% of the time. We see similar splits the other direction at 45% and 55% odds, with 55% predictions happening 41% of the time and 45% predictions happening 58% of the time. These are not great predictions, and statistical modeling does not seem to give much of an advantage here over talking to an experienced canvasser, for example. In fact, if I placed bets with odds-based payouts against every event that FiveThirtyEight gave between 45% and 55% odds of happening, I would make a ton of money off of them. Similarly if I placed bets with odds-based payouts against events with <=40% chance of happening or for events >=60% chance, I would also win a lot of money.
FiveThirtyEight uses other metrics like Brier Score to evaluate their models, and they do seem to substantially outperform a very naive model, but my point here is that they do not necessarily outperform an experienced campaign worker simply using their historical knowledge without the bells and whistles of a complicated model. They may not even outperform a random person who is given the election results from the last election cycle and asked to predict this cycle. And this is an issue that’s pervasive in both politics and business. Some might call this analysis paralysis, but I think it’s a slightly different animal. Bad decisions are made all the time based on models and studies that have an air of rigor to them, but under the hood they aren’t much better than asking a seasoned expert. Moreover, they may be missing the mark entirely because they fail to account for factors not included in the study or in the modeling data. But since the study or the model is labeled as “science” it is given heft that it may not deserve. This is the foundation of scientism.
I will not rehash it all here, but we saw a lot of this with COVID policy from the very beginning, and we have not yet reckoned with how scientism in the pandemic damaged trust in real science. We see it all the time with “market research” and forecasting. We see it in cash flow analysis, because nobody knows what the interest rates will be in three years. It all boils down to putting too much faith in an analysis that has a ton of uncertainty baked into it. This is not to say that studies and models are not useful, but it is to say that we all need to get much better at communicating where there is uncertainty, and how that uncertainty might manifest.
I appreciate Silver’s statistical rigor, and how he brings that to discussions that typically lacked much rigor. But Silver spends a lot of time evangelizing the data, and frankly it is good, but not that good.